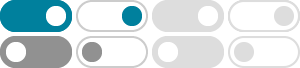
What is the difference between a convolutional neural network …
Mar 8, 2018 · A CNN, in specific, has one or more layers of convolution units. A convolution unit receives its input from multiple units from the previous layer which together create a proximity. Therefore, the input units (that form a small neighborhood) share their weights. The convolution units (as well as pooling units) are especially beneficial as:
machine learning - What is a fully convolution network? - Artificial ...
Jun 12, 2020 · The typical convolution neural network (CNN) is not fully convolutional because it often contains fully connected layers too (which do not perform the convolution operation), which are parameter-rich, in the sense that they have many parameters (compared to their equivalent convolution layers), although the fully connected layers can also be ...
In a CNN, does each new filter have different weights for each …
Typically for a CNN architecture, in a single filter as described by your number_of_filters parameter, there is one 2D kernel per input channel. There are input_channels * number_of_filters sets of weights, each of which describe a convolution kernel. So the diagrams showing one set of weights per input channel for each filter are correct.
When training a CNN, what are the hyperparameters to tune first?
Firstly when you say an object detection CNN, there are a huge number of model architectures available. Considering that you have narrowed down on your model architecture a CNN will have a few common layers like the ones below with hyperparameters you can tweak: Convolution Layer:- number of kernels, kernel size, stride length, padding
How to use CNN for making predictions on non-image data?
Feb 7, 2019 · You can use CNN on any data, but it's recommended to use CNN only on data that have spatial features (It might still work on data that doesn't have spatial features, see DuttaA's comment below). For example, in the image, the connection between pixels in some area gives you another feature (e.g. edge) instead of a feature from one pixel (e.g ...
How do I handle large images when training a CNN?
Aug 31, 2017 · Commonly researches scale the images to a resonable size. But if that's not an option for you, you'll need to restrict your CNN. In addition to downsampling in early layers, I would recommend you to get rid of FC layer (which normally takes most of parameters) in favor of convolutional layer. Also you will have to stream your data in each epoch ...
deep learning - Can I build a CNN for image classification tasks …
Theoretically, you should be able to stitch everything together into a complete CNN. However, I have not used any of those, and I have no idea about the level of maturity of the implementation. That said, if you are willing to implement a custom CNN from scratch, you will probably get more control over the implementation using a generic (BLAS ...
neural networks - How do we combine feature maps? CNN
Nov 21, 2022 · In Convolutional Neural Networks we extract and create abstractified “feature maps” of our given image. My thought was this: We extract things like lines initially. Then from different types of lin...
convolutional neural networks - How is parameter sharing done in …
Jun 18, 2021 · The same features might be across any location in the image, for a face recognition model, if the data is not very good it might happen that along with learning the features such as eyes and lips it also learns their locations if we only apply the convolution operation at a specific locality.
Is there any difference between ConvNet and CNN?
Feb 15, 2021 · I don't believe there is any particular reason to choose one over the other: ConvNet is slightly easier to say out loud and CNN is slightly shorter to write, but there is absolutely no difference in meaning. For some contrasting examples in the literature, the EfficientNet paper chooses the term ConvNet and this paper on AlexNet chooses CNN ...